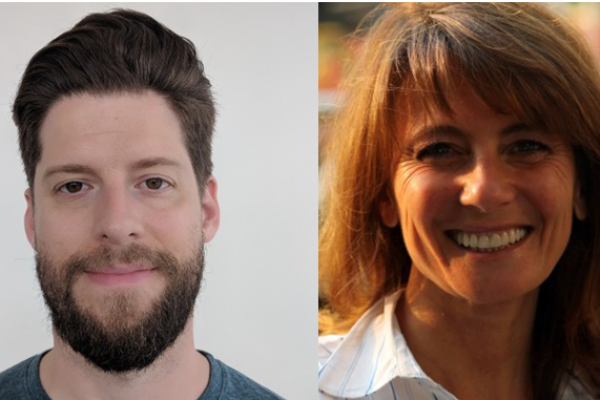
UBC Computer Science Master’s Student and Professor Awarded for Bringing Clarity to Eye-tracking Data
For MSc student Shane Sims and Professor Cristina Conati, both of UBC Computer Science, winning Best Papers together seems to be a pattern.
Their first award came in 2019, when Shane, fellow MSc student Vanessa Putnam, and Professor Conati won Best Paper at the IJCAI Workshop on Humanizing AI for Detecting Confusion from Eye-tracking Data with Recurrent Neural Networks.
Then, this year in October, Shane and Cristina were awarded Best Student Paper in the 22nd ACM International Conference on Multimodal Interaction for their paper: A Neural Architecture for Detecting User Confusion in Eye-tracking Data.
Encouraged by recent success with deep learning, they investigated the effectiveness of a novel application for detecting confusion amongst users viewing a screen, leveraging eye-tracking data. Using a novel architecture to combine Recurrent Neural Network (RNN) and Convolution Neural Network (CNN) sub-models in parallel, they were able to take advantage of the temporal and visuospatial aspects of data. The model they developed outperformed an existing model by 22% in combined ‘confused’ & ‘not-confused’ class accuracies.
“Shane was very passionate about his work,” said Cristina. “It was exciting to collaborate with him as he was always developing new ideas. If anything, I almost had to curb his enthusiasm!” she laughed.
Shane was honoured to win the ACM paper. “It was very much a surprise to get the 2020 Best Student Paper Award. In fact, I was surprised we were even nominated! I was only a Master’s Student, whereas others were PhDs or postdocs. It sure is a nice way to finish up my Master’s Degree.”
Eye-tracking data has become used more widely in AI for real-time predictions using factors like pupil dilation, proximity to screen and the direction of scanning. “I am getting two more eye-trackers to be able to continue this research,” Cristina said.
Congratulations go out to both on their award. Professor Conati and Shane have indeed created a new vision for the future of eye-tracking, using the robust capabilities of Machine Learning.